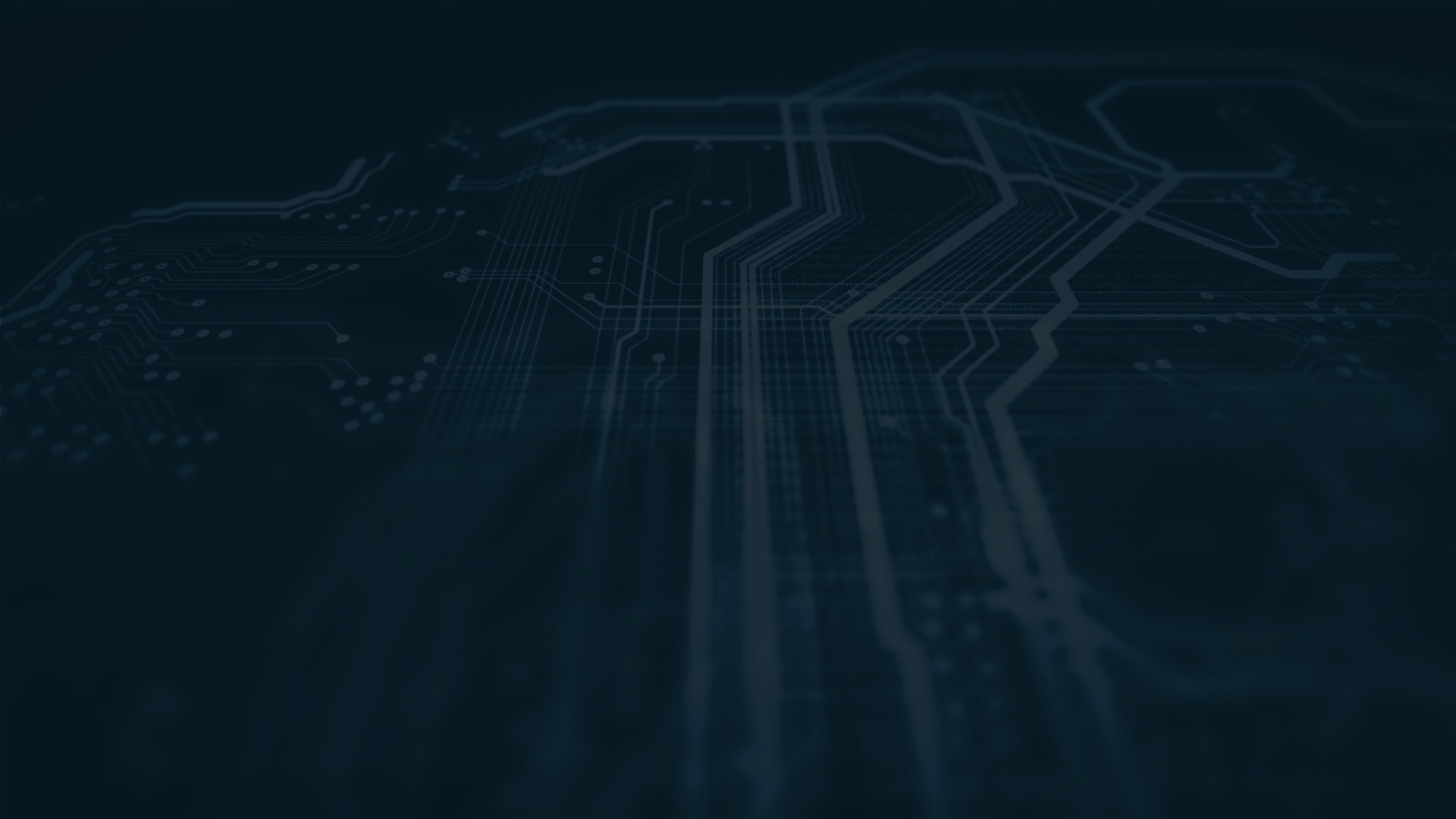
WikiPhish: A Diverse Wikipedia-Based Dataset for Phishing Website Detection
Gabriel Loiseau, Valentin Lefils, Maxime Meyer, Damien Riquet
Hornetsecurity
Hem, France
CODASPY2024
Abstract
Phishing remains a pervasive security threat, necessitating effective and universally comparable detection systems. The use of supervised machine learning models for phishing detection has been generalized in the literature to automate predictions and increase the detection capacities of security systems. These models rely on large amounts of annotated data for their training, evaluation and maintenance. Thus, there is a need to efficiently collect significant amount of annotated data to improve phishing detection. This paper introduces WikiPhish, a novel, renewable, and open-access dataset for phishing website classification. It consists of 110,606 webpages harvested from URLs drawn from Wikipedia’s references and the popular phishing databases OpenPhish and PhishTank. The dataset is designed to address the challenges of phishing detection by leveraging Wikipedia’s contribution verification and wide-ranging content. WikiPhish offers a more diverse and robust baseline for developing phishing detection models. We highlight the importance of gathering diverse URLs for building phishing website datasets, and demonstrate the practical utility of WikiPhish by employing it in the training and evaluation of phishing detection machine learning models.
Welcome to the website related to the paper “WikiPhish: A Diverse Wikipedia-Based Dataset for Phishing Website Detection”, published in proceedings of the 14th ACM Conference on Data and Application Security and Privacy (CODASPY 2024). This website contains informations about the dataset and the form to request access to it
Overview
WikiPhish is a new dataset for phishing website classification. The dataset comprises 87,563 legitimate web pages and 23,043 phishing web pages, including their URLs, HTML content, and a screenshot of the page. WikiPhish offers a diverse and robust benchmark for developing phishing detection models. The legitimate part of the dataset was created by exploring and filtering the “References” section of random Wikipedia pages. Data from Wikipedia was collected using the MediaWiki API. The phishing web pages come from the open phishing databases OpenPhish and Phishtank, collected from January 11, 2023, to October 22, 2023. These databases were used to obtain the latest phishing URLs that were published, reported, and verified by the community at that time. To limit redundancy in the legitimate and phishing documents, the number of appearances of the same Fully Qualified Domain Name (FQDN) was limited to 10 items per FQDN. This restriction is applied independently to the legitimate and phishing documents in the dataset, ensuring that a public hosting service is neither overrepresented nor underrepresented in any class of the dataset. More information can be found in the paper.
Dataset Name | Dataset Version | Creation Date | Phishing Collection Period | Number of Benign Documents | Number of Phishing Documents |
WikiPhish | 1 | October 2023 | January 11 – October 22, 2023 | Number of Benign Documents | 23,043 |
Access
To obtain access to the dataset, you can contact Gabriel Loiseau (gabriel.loiseau@hornetsecurity.com).
Acknowledgement
We thank the CODASPY reviewers for their helpful feedback and comments.
BibTeX
@inproceedings{10.1145/3626232.3653283,
author = {Loiseau, Gabriel and Lefils, Valentin and Meyer, Maxime and Riquet, Damien},
title = {WikiPhish: A Diverse Wikipedia-Based Dataset for Phishing Website Detection: Data/Toolset Paper},
year = {2024},
isbn = {9798400704215},
publisher = {Association for Computing Machinery},
address = {New York, NY, USA},
url = {https://doi.org/10.1145/3626232.3653283},
doi = {10.1145/3626232.3653283},
booktitle = {Proceedings of the Fourteenth ACM Conference on Data and Application Security and Privacy},
pages = {361–366},
numpages = {6},
keywords = {datasets, machine learning, phishing website detection, web security},
location = {Porto, Portugal},
series = {CODASPY '24}
}